What is representative data in product intelligence?
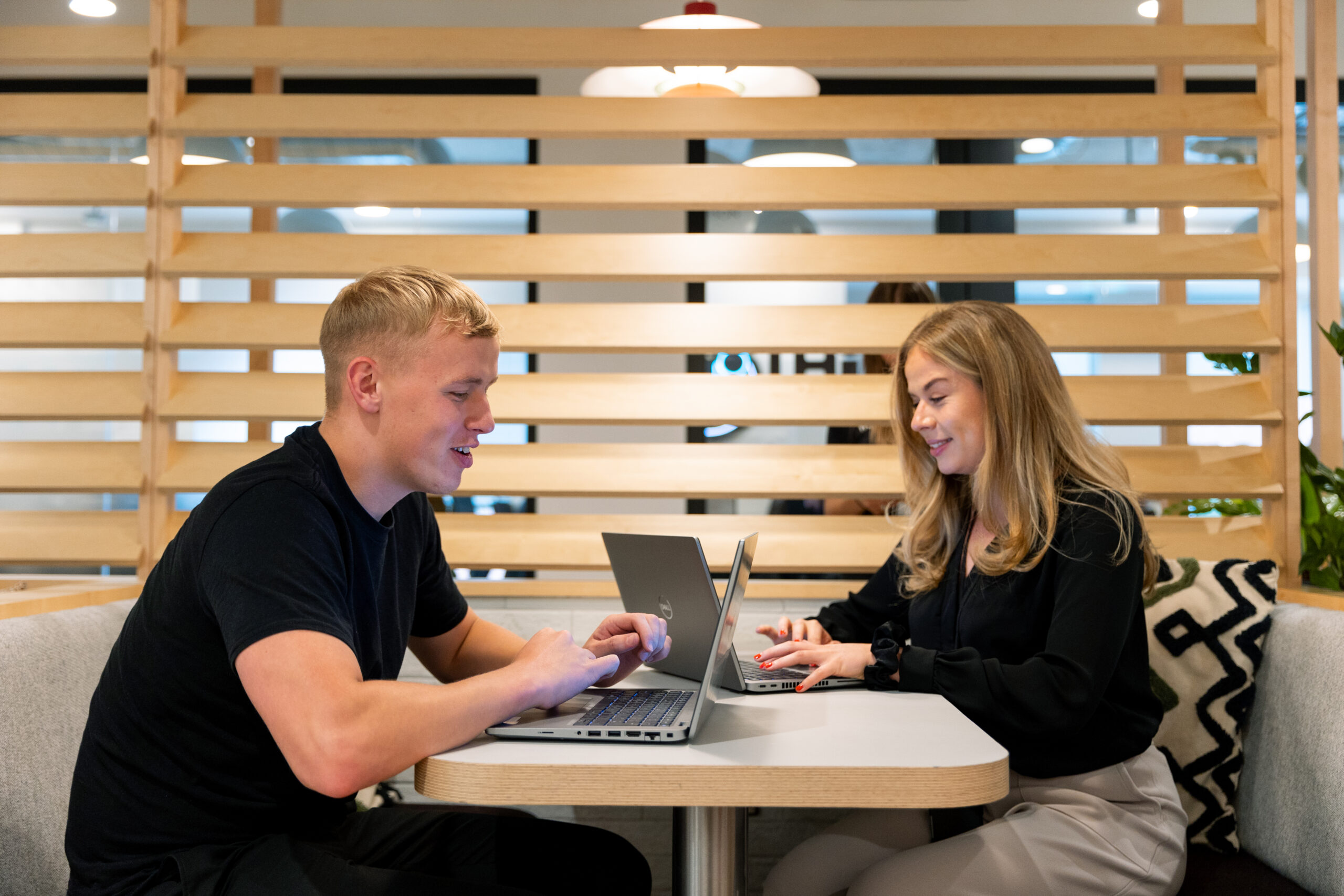
Actionable insights let you make better product decisions to increase your business ROI. However, to collect reliable data, you need a sample group that accurately represents your target audience.
Continue reading to explore the question, “What is representative data, and why is it essential for product intelligence?”
What is a representative data sample?
Both quantitative and qualitative research studies involve collecting data from target demographics. Samples allow you to divide a whole population into more manageable versions.
Generally, a larger sample size can help reduce sampling error. However, when working with large demographics like an entire country, you can’t always include every single member. So what does representativeness mean in terms of data sampling?
Using a sample that accurately reflects your target population helps you represent a specific group without including every single member. A reliable sample is representative of key characteristics like age, gender and socioeconomic status. Whether you conduct surveys or interviews, a representative sample lets you collect data and gain insights for data-driven decisions that truly reflect your target audience.
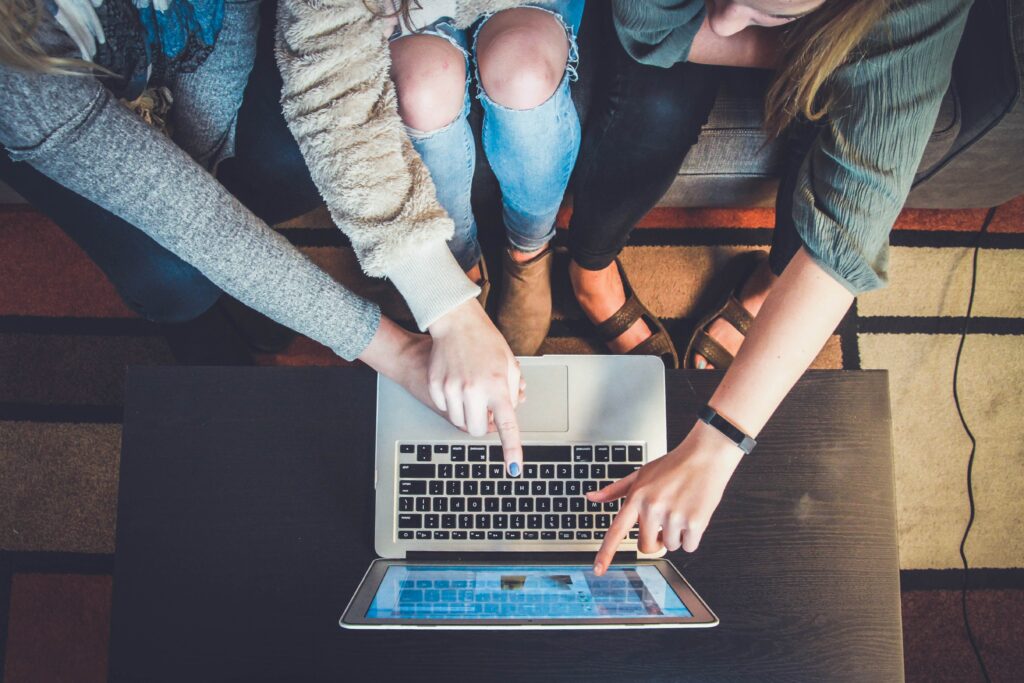
What does a representative sample mean for product intelligence?
Representative sampling has several key benefits for product intelligence processes. A truly representative sample is important for ensuring data quality standards in consumer and market research.
Researchers can use representative samples to reduce bias and avoid sampling errors. Accurate, unbiased representation leads to more reliable consumer intelligence data. This is a key element of statistical analysis that results in actionable insights for improved product development decisions.
Creating a representative sample
Your sampling methodology will impact the representativeness of your final sample. When
determining the best sampling method for your needs, you should consider factors like the size and characteristics of your target audience.
Representative sample methods revolve around probability sampling (random sampling) and non-probability sampling (non-random sampling). A probability sampling method helps avoid sampling bias, as all population members have an equal probability of being selected for a smaller sample group.
Common representative sampling methods include:
- Simple random sampling: Selecting sample participants arbitrarily.
- Systematic sampling: Select sample participants at regular intervals.
- Stratified sampling: Selecting random sample participants from pre-defined subgroups (e.g., age brackets).
- Quota sampling: Select non-random sample participants from pre-defined subgroups.
Maintaining data representativeness
It’s important to consistently evaluate your sampling methods to accommodate evolving consumer and market trends. You may need to update your selection process to accurately reflect new target audience profiles and perspectives.
At Vypr, we continuously evaluate our community members with KPIs like Quality of Fit. This compares active users against the latest population profiles to ensure they accurately reflect larger group demographics.
Vypr: Representative consumer and product intelligence
Vypr is the world’s leading product intelligence platform that enables businesses to rapidly understand changing consumer behaviour through fast, cost-effective consumer insight.
It’s not enough to understand, “What is representative data?” Product success demands representative sampling for actionable insights. Vypr’s platform offers advanced customer segmentation for accurate results.
Access reliable product intelligence data with representative sampling methods. Try Vypr free now.