What is data reliability in product intelligence?
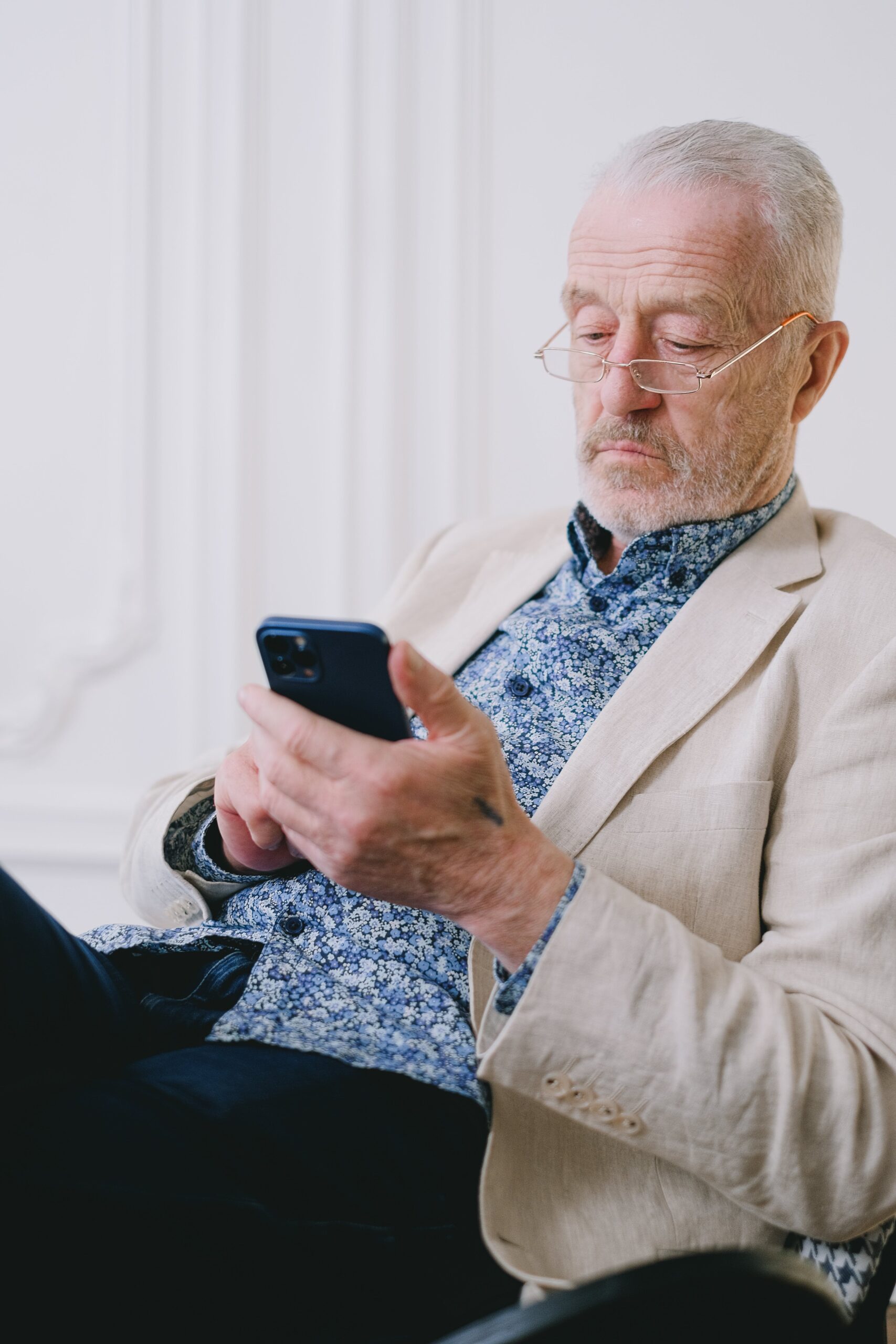
Consumer data is the foundation of any product intelligence tool. However, data quality plays a major role in influencing the effectiveness of product development and improvement processes. Avoiding unreliable data is essential for ensuring product and business success.
Continue reading to discover the answer to the question, “What is data reliability, and why is it important for businesses?”
What is data reliability?
Data reliability refers to how trustworthy a dataset is based on its completeness and ability to be both accurate and consistent in its results. Organisations can use the insights gained from the analysis of reliable data to make informed decisions. These generally revolve around strategy, resource allocation and other business operations which facilitate success.
The role of data reliability in product intelligence solutions
Product intelligence involves the collection of reliable customer data to better understand how consumers perceive, use and respond to a particular product. Such comprehensive data includes product analytics, user behaviour and more.
This information is carefully analysed to uncover actionable insights that inform product development and marketing tactics across multiple teams. These data-driven decisions are undertaken with the goal of increasing customer satisfaction and product success. However, the results of this process depend on the reliability of the initial product data intelligence.
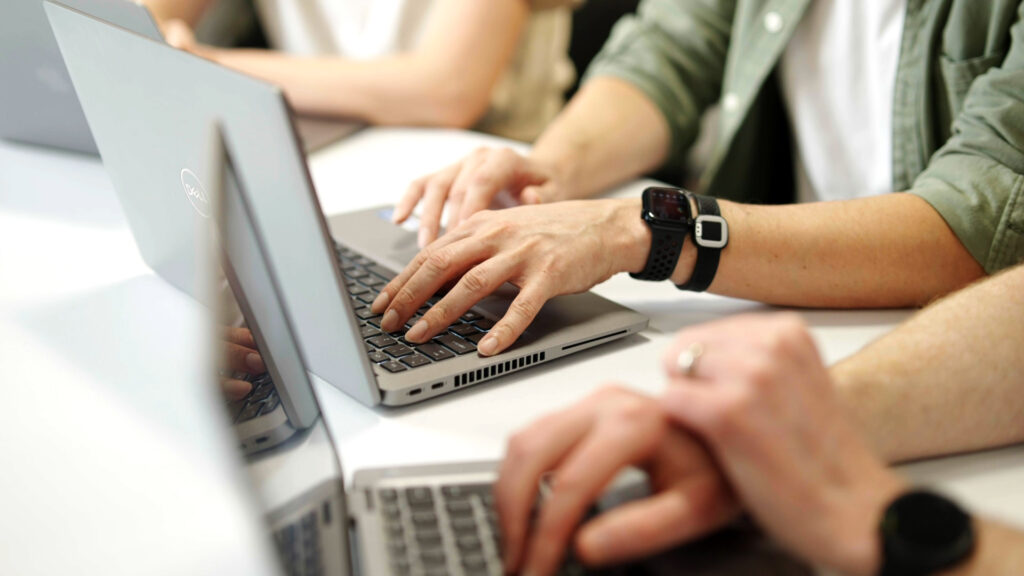
Benefits of reliable data for product intelligence
Reliable customer data is key to any successful product intelligence undertaking and results in numerous business advantages. Exploring these benefits will help you understand the importance of the validity and reliability of data used within research and decision-making processes.
Valuable insights
The collection of accurate data enables you to gain meaningful insights into consumer needs, wants, behaviour, perceptions and preferences. This helps create a more complete picture of who your consumers are and how your products can cater to them in the most effective way possible.
Improved decision-making
Customer data plays an important role in influencing business decisions. An increase in data validity and reliability provides you with more robust consumer insights. This knowledge helps you make better decisions regarding product development and enhancement.
By analysing feedback from product analytics tools, you can identify opportunities for improvement in existing product designs. You can also test new ideas with your target audiences before implementing them. This facilitates effective decision-making based on data rather than assumptions and results in happier customers.
Customer and product success
The true test of any product is how consumers respond to it. Access to reliable intelligence enables you to implement robust data-driven decisions throughout every stage of the product development life cycle. In turn, this results in better customer experiences and overall product success.
Better ROI
Ensuring data reliability can benefit your business ROI in several ways. By taking advantage of actionable consumer insights and continuous product validation testing, you can create a product that is expertly tailored to your target audience. This will help you avoid expensive changes towards the end of your development processes. It will also help increase customer engagement and bolster trust in your brand.
Not only does this save money and increase sales, but it also boosts customer loyalty and retention for a combination of short- and long-term ROI. You can monitor these achievements by tracking product development metrics like customer lifetime value.
Competitive advantage
Continuous collection and evaluation of reliable product analytics and customer feedback ensures you stay up-to-date with ever-changing product and market trends. Remaining at the forefront of these trends helps enhance your relevance and product lifespan for a distinct competitive edge within your field.
Drawbacks of unreliable data for product intelligence
Unreliable data not only prevents you from reaping the full benefits of product intelligence, but it also results in several other interconnected disadvantages.
Here are some of the most common drawbacks of unreliable and invalid data.
- Incorrect conclusions. By analysing inconsistent data, you will inevitably gain inaccurate consumer insights. These insights lead to incorrect conclusions about how to solve an issue or improve a product, resulting in decreased efficiency.
- Poor decision-making. Without valuable insights on which to base your decisions, you are forced to rely on inaccurate data or assumptions for guidance. This results in poor and ineffective decision-making processes.
- Negative customer experience. Flawed product development decisions result in end-products that fail to meet consumers’ expectations, wants and needs. Such unsatisfactory offerings will negatively influence customers’ experiences and trust.
- Decreased ROI. Data reliability issues directly correlate to a decrease in business ROI. Customers will not buy unsatisfactory products or stay loyal to the brand that produces them. This leads to a decrease in sales and customer retention rates that reduce short- and long-term ROI.
Factors that can impact data reliability
Now that you have an overview of the advantages and disadvantages of data reliability, it is key to explore what makes data reliable or unreliable and how you can control this. Ensuring the reliability and validity of a data collection is a continuous process that requires consistent effort and evaluation.
Key influences include the sources, scope and accuracy of your information. Understanding these factors will help you avoid data quality issues so that you can get the most out of your product intelligence.
Data sources and collection methods
The reliability of data is greatly dependent on where it is sourced from. Unreliable sources are more likely to provide data that contains errors and biases. For the most credible information possible, it is best to use a trustworthy data source that is well-known for providing accurate data. Popular options for this are educational and government institutions.
However, even if you’re using a trusted source, it is still important to verify your data. You can do this by referencing information across multiple credible sources.
The ways in which customer data is collected also have a major impact on its overall reliability. For example, surveys are at risk of using sample groups that do not accurately represent the targeted real-life audience. Such biases result in flawed, unreliable data. Given this, it is essential to rely upon collection methods and intelligence tools that have been tested to ensure they produce reliable data.
Data consistency
Within the sphere of data, consistency refers to information that remains the same across different platforms like company databases. This is an essential way to improve data reliability, as it ensures your data will produce the same results when tested in different contexts for maximum accuracy.
Having inconsistent data can lead to conflicting results that make it difficult for you to effectively identify product development issues. This can result in ineffective decision-making and a decrease in customer trust.
Error management
No matter how refined your data collection methods are, it is inevitable that there will be some errors within every dataset. These can include everything from the misinterpretation of information to an incorrect data entry. Given this, it is no surprise that there is a connection between the quantity and type of errors within a dataset and its reliability.
However, instead of simply attempting to reduce errors, effective error management techniques also involve accepting and accounting for the presence of errors within a dataset. By acknowledging that errors exist, you can implement effective strategies to identify and correct them. You can also use error margins to account for any inconsistencies or mistakes you may miss. These combined efforts help to manage potential errors and ensure data reliability and validity for accurate analysis.
Shifts in consumer trends
When collecting data over a long period of time, it is essential to be aware of potential shifts in consumer trends. Even though data may have been reliable when it was originally collected, changes in market trends and consumer behaviour mean it can easily become unreliable at a later stage. To effectively account for these changes, you should consistently re-examine and compare datasets to identify and correct inaccuracies.
By not looking out for changes over time, you risk the use of outdated and unreliable data in current decision-making processes. However, it’s also important to note that older data is not always automatically less accurate than newer data. Rather, it may fluctuate in accuracy over time. This can be dependent on external influences such as seasonal changes.
For example, consumer interest in winter clothing is likely to be higher during cold weather months. While data based on this higher interest level would be useful during winter, it may not be applicable during summer.
Data comprehensiveness
For maximum reliability, a dataset should be comprehensive enough to provide effective and valuable insights about your target market. This process includes examining a diverse and representative sample group, as well as accounting for potential key variables that may influence findings.
Biases are another common characteristic of bad data. To avoid biased findings, a dataset should be comprehensive enough to allow for the generalisation of statistical insights within broader contexts.
Best practices for maintaining data reliability
There are several techniques for ensuring the validity and reliability of research data. Implementing these methods enables product managers and designers to continue making informed decisions for business success. Essential data reliability initiatives revolve around the concepts of data observability and standardisation.
Data standardisation
The standardisation of data applies to everything from gathering new data to formatting entire data governance systems. It involves implementing precise data governance frameworks and policies to systematically manage how data is collected, stored and accessed.
Standardised data practices are extremely useful for organising and keeping track of large quantities of data. They facilitate quick and easy comparisons across datasets to help validate information and identify any inconsistent or incomplete data entries.
Try Vypr for Free
Data observability is a broad concept which involves consistently monitoring the health of your data through processes such as data audits, comparisons, analyses and more. Consistent and in-depth monitoring facilitates the easy identification of bad data.
By pinpointing data quality issues, observability methods enable you to quickly and effectively resolve them for increased data validation and reliability.
Make better decisions faster with Vypr’s product intelligence platform
Vypr is the world’s leading product intelligence platform that enables businesses to understand changing consumer behaviour through fast, cost-effective consumer insight.
Ensuring data reliability has never been easier than with Vypr’s robust data collection platform. You can effortlessly collect both qualitative and quantitative data from the trustworthy Vypr Community.
Enjoy easy access to comprehensive, reliable data with Vypr. Book a demo now to find out more.